Integrating AI Diagnostics in a HIS
2023 will undoubtedly go down as “the year of AI”. It all started with ChatGPT, but every major company is now working on some AI application in their field. In healthcare, however, this process has been going on for some years now. And it is at the stage where it can be used to diagnose medical imaging or video, looking for anomalies.
AI can help in medical imaging diagnostics
Analyzing medical images, like X-Rays, MRI or CT Scans, as well as ultrasound images or videos is hard. That is why it takes a lot of training to become a proficient radiologist, with the ability to spot changes in normal human tissues and make correct diagnosis. But, as with many other fields, artificial intelligence has also arrived in the medical sector. It is able to do a lot of things, as we are seeing in this trilogy of articles, but one of the most interesting is the possibility of doing diagnostics in medical imaging.
A properly trained AI model, that has been shown hundreds or thousands of digital medical images and videos, like those from modern X-Ray machines, or MRI, CT Scan or PET scans, can be able to spot anomalies in the tissues. Sometimes even those small anomalies that a human would not notice or see.
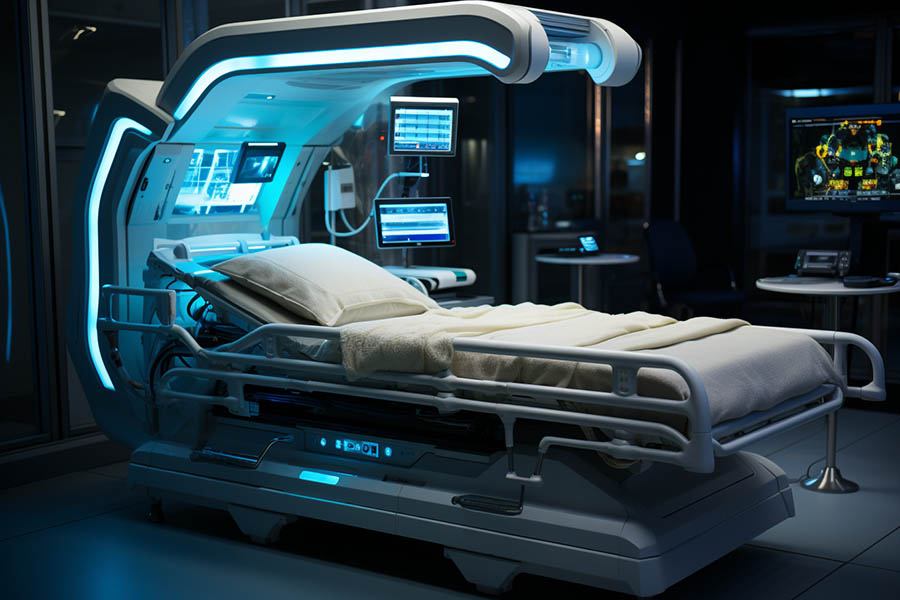
Early warning with AI
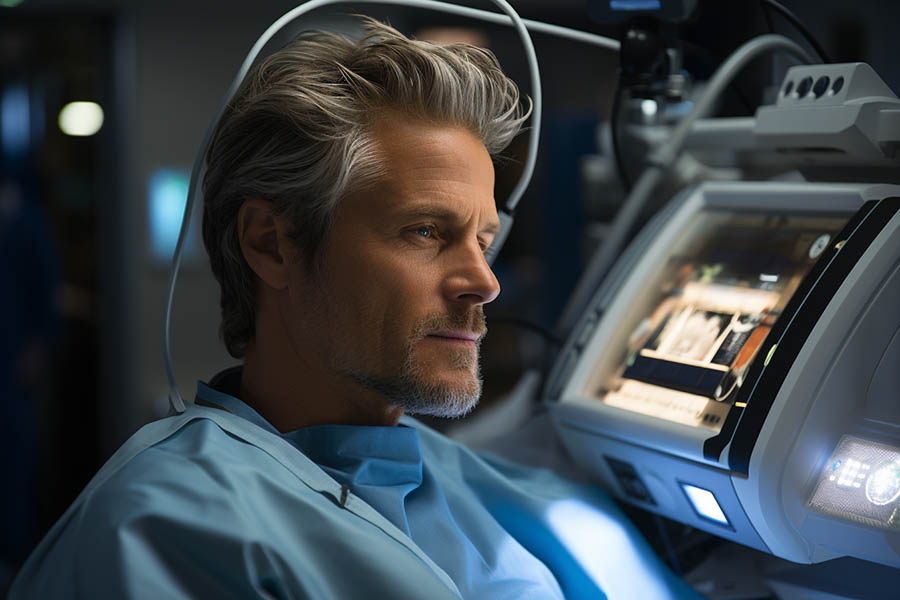
But why should this be important? Isn’t an experienced human radiologist better than any AI. Yes, he or she is. But, and there is a ‘but’ here, if the changes are small, the human eye, however experienced, may not notice them or dismiss them. This means that the artificial intelligence image analysis, which has received many thousands of images, could to a diagnostic or prognosis quicker and earlier than the human counterpart. And this, in turn, can help save lives. The beginning of a tumor or other anomaly, spotted by an AI algorithm before a human radiologist, can instigate further investigation by the medical professional, like additional tests or bloodwork. And this could help finding the beginning of an illness than then can be treated earlier, with a higher success rate.
What else can a medical AI help with?
Actually, almost with everything. The fact that an AI algorithm has to be trained first to be effective, is the key point here. Just like a doctor has be trained in different areas, the same can be done to an AI. In fact, an AI can be trained far more specifically than any human could, because all the AI models rely on two things: the model itself, and the data it has been trained on/is still being trained with each new patient. This allows for a kind of specialization that is difficult to achieve as a person. We can’t deal with immense amounts of data as easily as a machine can. But artificial intelligence has much more application than only image diagnosis. Here are some of them:
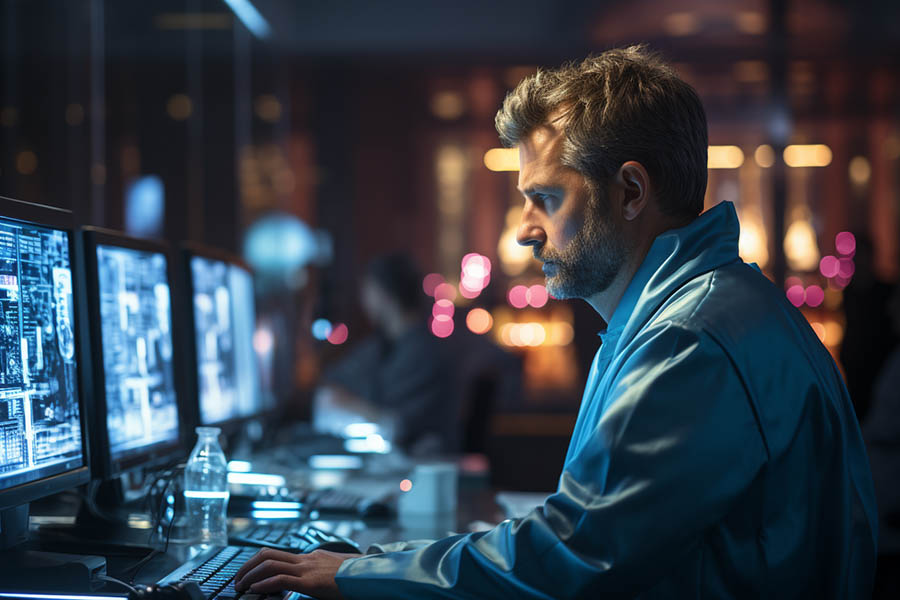
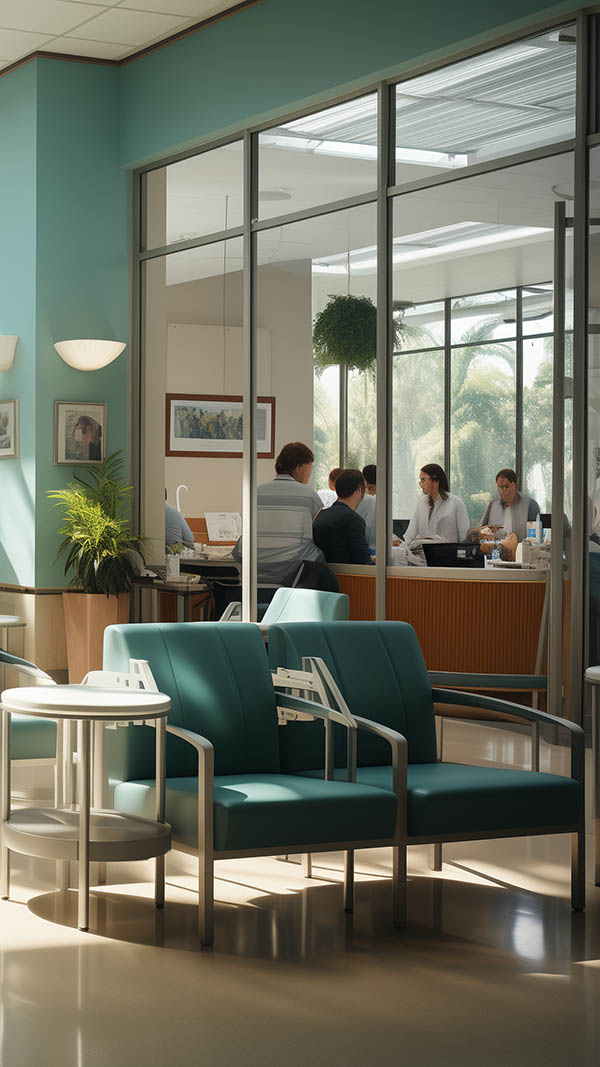
- Image Analysis and Interpretation. That’s the one we’ve been talking about and is only here to complete the list. AI algorithms can identify and outline abnormalities, tumors, or specific structures in medical images, helping radiologists in their analysis giving a “second opinion”.
- Quantitative Analysis. AI can provide precise measurements and quantitative data from medical images, aiding in the assessment of disease progression and treatment effectiveness. This can be very useful in medical research, for example.
- Early Warning Systems. We’ve also talked about these. The precision of an artificial vision system can surpass human abilities and detect anomalies much smaller than a person, thus raising an alarm, and starting treatment earlier.
- Tailored treatments. Artificial intelligence is able to devise personal treatment plans based on all the patient’s information, and the success rates of different drugs on similar patients, taking into account individual variations in anatomy and pathology.
- Automation. Routine or repetitive tasks, such as image preprocessing and quality control, can be automated, saving time for healthcare professionals, who can do higher value work.
- Workflow optimization. AI can enhance the speed of image analysis and interpretation, leading to faster diagnosis and treatment decisions. Besides, the algorithms can improve the quality of medical images, making it easier for healthcare professionals to interpret and diagnose.
- Reducing workload. By automating routine tasks (as seen in point 5), AI can reduce the workload on healthcare professionals, allowing them to focus on more complex cases and spend more time with patients.
- Decision Support Systems. Very close to the “second opinion” topic, AI can serve as a tool for radiologists and surgeons, providing additional insights and offering recommendations based on its analysis of hundreds of similar medical images.
- Training and Education. Although we are going to see a take on this in part III of this series, AI can be used in educational settings to simulate and generate medical imaging scenarios for training purposes, allowing healthcare professionals to enhance their skills.
Conclusion
In summary, AI in medical imaging, like our own Videomed contributes to more accurate diagnoses, improved patient treatments, and increased efficiency in healthcare workflows. One very important point is that AI is not going to substitute medical professionals, but complement them. We are still at the beginning and new fields and uses are going to be discovered in the upcoming years.
In our last article in this series of three, we are going to have a look on surgical recordings and how they can be used not only for training doctors and nurses, but also for other purposes thanks to the intervention of AI. To see first part here.
Medical video in a PACS and a HIS
Digital Medical Imaging is an important part of the modern diagnostic process. Be it X-Rays, CT Scans, MRIs or ultrasound machines, all of them produce DICOM images today, so the doctors can see them on screen at any point. But the reality isn’t so rosy at all…
Format incompatibility
In today’s healthcare, medical imaging has an ever more important role when diagnosing a patient’s condition. The systems have gotten so advanced and have such good resolution, that diagnostics are made with more certainty than ever. But, in contrast to the imaging “of old” (where “old” is just a couple of years back), today most of the medical imaging devices generate digital files, instead of having to develop film or the like.
On top of that, most of them do not generate only static images, but also video files. For these images there is the DICOM format (Digital Imaging and Communications in Medicine) which is a standard protocol for the management and transmission of medical images and related data. In fact, DICOM also supports several video formats as well as multiframe images, like the ones in CT scans. However, this is where the problems arise. Most vendors have a particular interpretation of their image formats, in order to lock the hospital to that particular brand, avoiding too much compatibility with the rest of the competing vendors.
The resulting scenario is one in which the medical professional cannot always use a PACS (Picture Archiving and Communications System) to access the images from his or her office, but has to go physically to the machine in question that did the images, and watch them there. The fact that DICOM uses no compression in the images to avoid artifacts (that could be misleading the doctor or radiologist) is one of the reasons. When recording video this also intensifies, as these files are so huge, that they usually cannot be transmitted over the hospital network in an efficient way. Hence the doctor has to go to the machine and watch the exploration video in situ. And this is far from ideal.
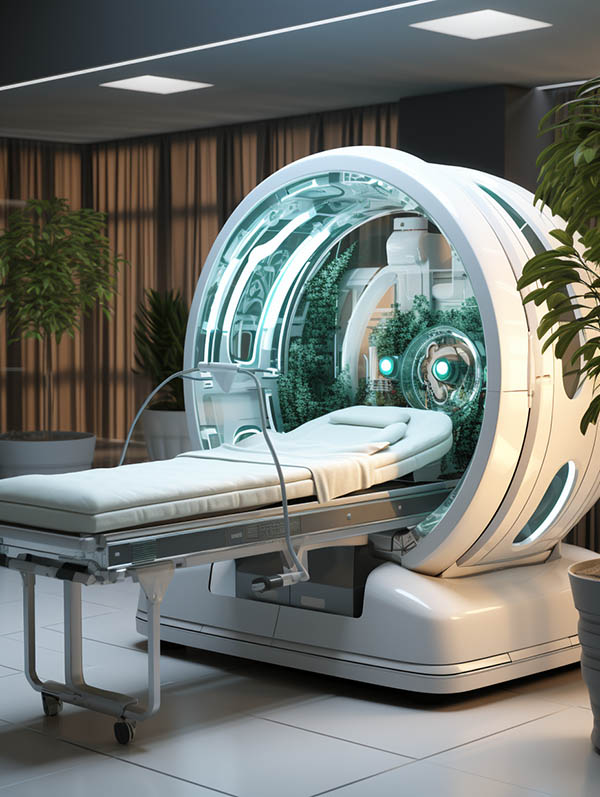
Information scattering
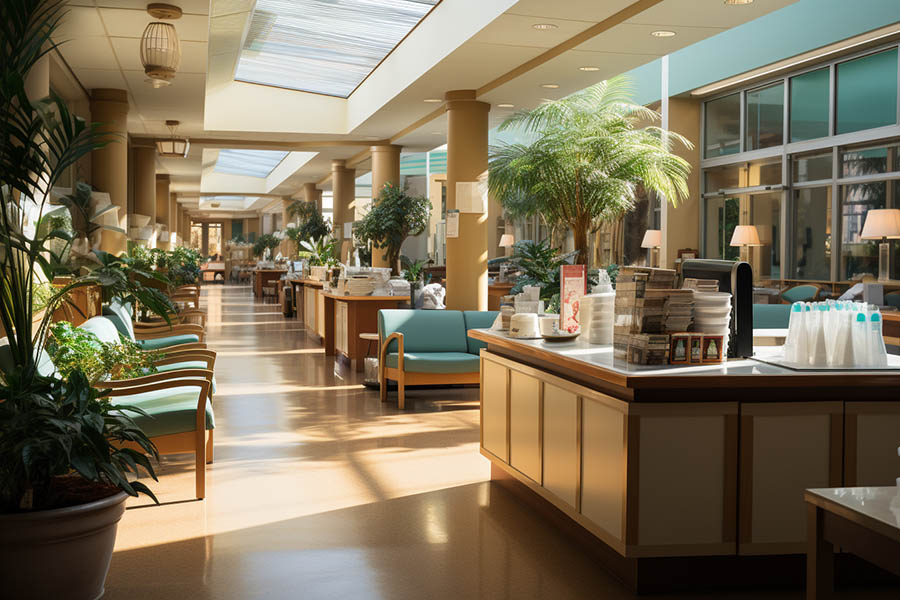
This situation leads to losing an enormous amount of time when doing one simple diagnostic, especially if the hospital is big. If you multiply this by all the patients that have imaging done, and the doctors that have to run around the hospital to gather all the information they need in order to make an accurate diagnostic, is it easy to see that this information scattering is not only costing time, but also money. And that the medical care quality suffers greatly under it.
The solution for DICOM images is usually the aforementioned PACS, which can be accessed from any computer in the hospital (or outside of it, depending on the security rules enforced). But most PACS do not support video directly, for a variety of reasons, chief among which is the file size and different formats. Hence the information scattering continues, because some data is in the HIS (patient data), some in the PACS (static images) and some on individual machines (medical video).
Images and video in the PACS
But there is a solution to this: adding a secondary video PACS can solve the problem of viewing medical video directly from the doctor’s office, without having to change the hardware or software in the hospital. It just needs to add the secondary video PACS, which integrates with the current PACS and, as we will see in a bit, also with the HIS. Videomed PACS is such a secondary video PACS.
What the secondary PACS does is gathering all the video from the machines by direct connection to the network and storing it centrally. Then all the formats are unified to a single one, usually a high quality MP4. This proxy file then can be viewed with ease and needing a low network bandwidth, from anywhere in the hospital, just be connecting to the PACS. If the doctor or surgeon needs to see the original file, this is also possible over a high speed network connection but, instead of involving the PACS, which may be used by all of the hospital constantly, the video is provided by the secondary PACS instead, taking load from the main PACS.
On top of this, the system anonymizes all the patient data for privacy and compliance reasons, so it never leaves the HIS or main PACS. And it is possible to specify retention periods for the videos (due to the space they take up in the system), so they are converted into lower resolution copies after a time. This makes for efficient storage, and keeps the system tidy, without losing any information.
And on top of this, platforms like Videomed PACS make use of artificial intelligence analyzers in order to catalogue the videos automatically, helping to speed the process up further, putting everything into place without human intervention. And, as we will see in part II of this series, it can integrate diagnostic AI analyzers as well, providing even more help for radiologists or surgeons, who can rely on a “second opinion” of the AI system.
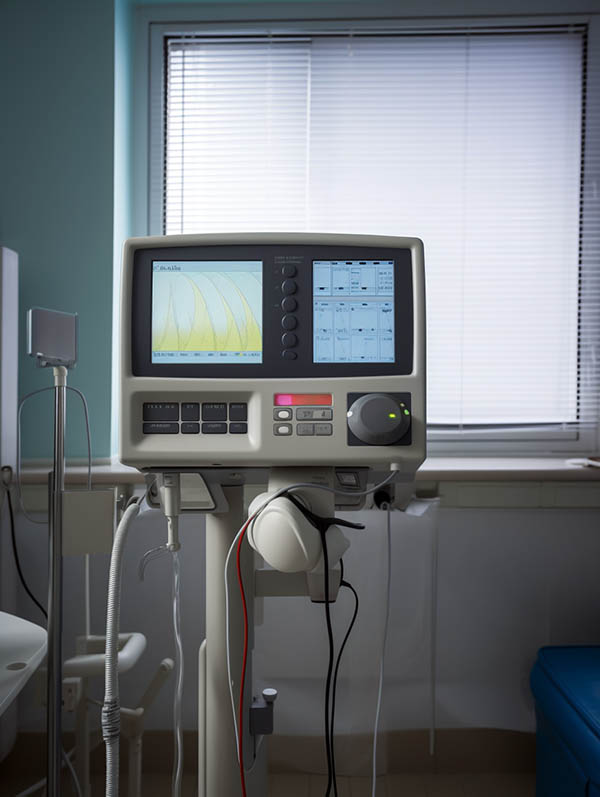
One central command point: the HIS
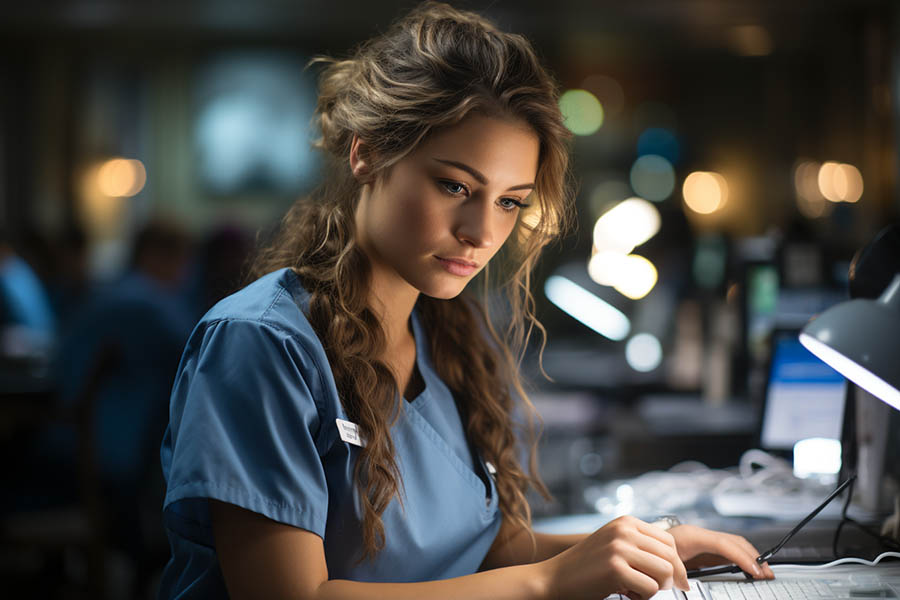
The use of a secondary PACS has another advantage: it can be integrated directly with the HIS, so all of the information can be retrieved together with all of the other patient record. In fact, with this system, you can integrate clips of important diagnostic videos directly in the HIS. The integrated viewer gets the data from the secondary PACS in a transparent manner, but it is all viewed inside the HIS, which is the central point for the doctors, so all of the information is on one screen, helping make better and quicker diagnostics. This way, the medical professional doesn’t have to walk through the hospital to gather everything he needs for a single patient, and can work much more efficiently.
Conclusion
To avoid scattering of medical video through a hospital or clinic, a secondary video PACS is the best solution, as it provides integration into the regular PACS, as well as the Hospital Information System, so the doctors can get all the diagnostic information they need in one place, all at once, and can work more efficiently, with all the data at hand.
In our next article in this series of three, we are going to talk about how AI Diagnostics are helping doctors and surgeons make better and quicker diagnostics. We’ll conclude the series with a look on surgical recordings and how they can be used not only for training doctors and nurses, but also for other purposes.
AI Analytics conquers healthcare
The introduction of AI in the healthcare sector has been going on for some years now. But recently it has taken on another dimension, with the advances in artificial intelligence and the capabilities that Machine Learning has proven to have for the medical profession. Advances in diagnostics, treatments and patient monitoring are helping to establish AI as a driving force for modern healthcare.
Better diagnostics & research
Doctors all over the world have to do the same task over and over again: observe the patient’s symptoms, have a look at the possible tests and use their experience and knowledge to find a diagnostic that fits it all, in order to decide on a treatment. But what if there was a quicker way to find a diagnostic, with more information, beyond the knowledge of the MD?
Image diagnostics with AI
Today, when most of the medical imaging is done digitally it was only logical that someone would come up with the idea of having AI analyze the images to make a diagnostic. Especially when an AI can have access to hundreds or thousands of images to compare them and draw conclusions.
Machine Learning, with access to large amounts of clinical documentation, can do what machines are good at: identify patterns and, possibly, make predictions about treatment outcomes or the evolution of the patient’s illness or recovery. This is not only an advantage for the Medical Doctors, but for the whole health sector, as AI could select the best treatment right from the start and save money or time.
Even if the radiologist (or similar) would have to check on the proposed diagnostic and treatment and give his approval, this simplifies the process and saves time. Especially if the AI is right. And, if it’s not, it will learn from the error for the next time.
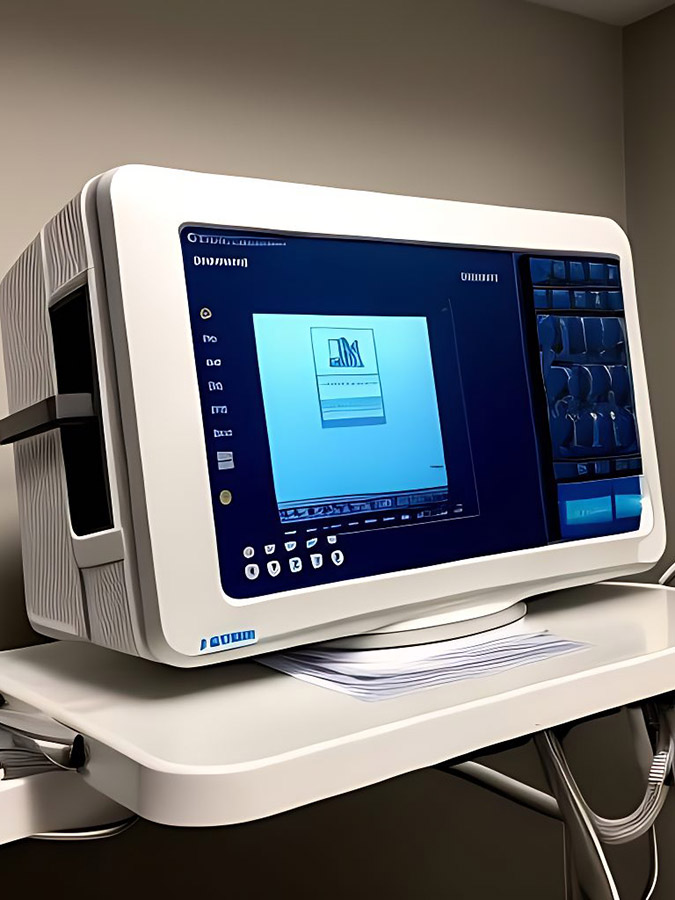
A smarter HIS

One of the main problems currently is that the different medical images reside in different systems or machines all over the hospital and the patient information usually is in the HIS (Hospital Information System). This difficults the analysis of all the available images, as they are not connected.
Many hospitals use a PACS (Picture Archiving and Communication System) but that presents another set of difficulties, like visualizing video recorded during explorations, MRIs, TACs or ultrasounds, which in many cases is not possible.
Our solution, Videoma Health bridges this gap and allows to integrate not only the PACS with all the medical imaging systems, of any provider, but to connect to the HIS/RIS to get all the patient information associated and, on top, provide the possibility of not only seeing recorded images, but also reproducing video, in any format or codec.
An enhanced Electronic Health Record
Almost all civilized countries use some form or other of an EHR (Electronic Health Record) for all of the patients. This database of information has an incredible value not only for a single patient, as the AI system can access all of his/her historical data to take into account for the current diagnostic, but if the access is global, the conclusions to be drawn for patients with similar symptoms or medical history is invaluable.
Of course, this may collide with privacy laws in many countries, but the benefits are clear. And even if the information is limited to the same hospital, many conclusions could be drawn regarding treatment successes, duration, and the like. In the end, the AI system would be able to make better recommendations to save more patients, shorten treatments or even find new ways of confronting a certain illness.
Impact on healthcare jobs
Obviously, every time when artificial intelligence or robots enter someone’s profession at first there is certain reluctance and mistrust. Humans don’t like to be displaced from their job. But, in this day and age, AI is entering most of the professions due to its capability of learning and of correlating really big data sets in very little time to arrive to conclusions. The overall short-term predictions is that AI in the health sector is not going necessarily to replace people, but be an additional tool that will help them make better diagnostics than before.
On the other hand, humans are not ready yet to trust their health to a machine, even if it is a very intelligent one. This, if it ever is going to happen, will take a good amount of time. However, having a “second opinion” from a machine that has analyzed hundreds or thousands of cases similar to the one at hand will be invaluable to define the most optimal treatment in many cases. And, as people become accustomed to this and the hit rate climbs, so will trust. So health care workers should not feel immediately threatened, but instead see AI as a new tool to use in their profession to do a better and, maybe, quicker job.

Conclusion
Artificial Intelligence and Machine Learning are taking a foothold in the medical and healthcare professions, to help make diagnostics, control treatments and, in general, give a second opinion based on hundreds or thousands of previous and similar data points, to reach the best conclusions, diagnostics and treatments.
Video recording and management systems in the health sector
Video recording and management systems in the health sector are increasingly being adopted in medical environments and organisations to improve patient care, streamline operations, and enhance safety. These systems allow medical professionals to record and store video footage of medical procedures, consultations, and other important events, as well as access and manage this footage in a centralised and secure manner.
One of the primary benefits of video recording and management systems in medical environments is their ability to enhance patient care. By capturing video footage of medical procedures, doctors and nurses can review the footage to ensure that they are following proper protocols and techniques. This can help to reduce the risk of medical errors and improve the quality of care that patients receive.
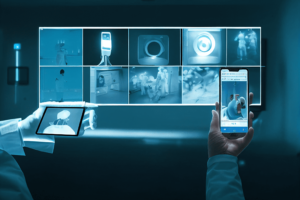
Medical Video recording and management systems can also help to streamline operations in medical environments. By providing a centralised repository for video footage, these systems can help to reduce the time and effort needed to locate and review footage. They can also help to improve collaboration between medical professionals by allowing them to easily share footage with one another.
In addition to improving patient care and streamlining operations, video recording and management systems can also enhance safety in medical environments. These systems can help to prevent incidents of abuse or neglect by providing a record of interactions between patients and medical staff. They can also help to protect medical professionals from false accusations by providing a clear record of events.
There are a number of different types of video recording and management systems available for medical environments and organisations. Some systems are designed specifically for use in operating rooms and other high-risk areas, while others are more suited to general use.
When selecting a video recording and management system for a medical environment, it is important to consider a number of factors. These include the system’s reliability, security, and ease of use. It is also important to ensure that the system is compatible with existing hardware and software, and that it can be easily integrated into existing workflows and processes.